The great power of AI in the business world is broadly recognized, yet many organizations still struggle with its deployment.
This challenge is especially true for large enterprises, where smooth integration across tech and business units can win or fail the corporate game. Gartner has revealed that slightly over 50% of AI projects make it into production, and these numbers were consistent for years between 2019-2022.
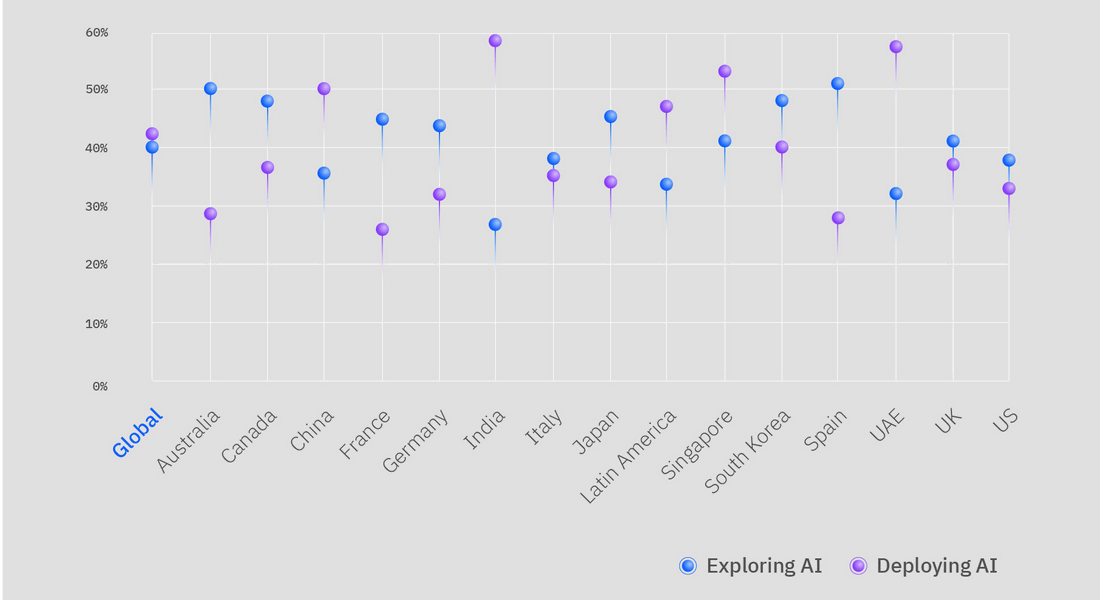
This is where AI orchestration comes into play.
AI orchestration is a practical approach to help companies develop a strategy for managing multiple AI tools and projects. Imagine a world where AI systems not only automate isolated routine tasks but create fully intelligent decision-making processes. This vision is not as distant as it may seem.
In this article, we'll explore the key concepts of AI orchestration, and its benefits, and review the top 5 AI orchestration platforms with their unique features and methodologies.
You'll also learn how to effectively use n8n, a source available workflow automation tool, for AI orchestration.
Let's dive in!
What is AI orchestration?
While the commonly accepted definition is yet to be specified, Pam Baker, in her insightful report on AI orchestration methodologies mentions the strategic coordination of AI technologies to manage various AI tools, processes, data, and talent within an organization.
The goal is to create an interconnected, intelligent decision-making ecosystem. This approach not only maximizes the business value of AI investments but also ensures that AI-driven initiatives are aligned with the company’s overall goals.
To understand the importance of decision intelligence, consider these real-world examples:
- Public health crisis (from the source mentioned above): During the COVID-19 pandemic, Dr. Anthony Fauci was able to share data about the spread of the virus, but struggled to make final decisions about safety in schools. True decision intelligence would bridge this gap, providing clear, actionable recommendations based on complex data analysis.
- Retail inventory management: A large retail chain could use AI to predict product demand based on historical sales data, seasonal trends and external factors like weather or local events. However, decision intelligence goes one step further by automatically adjusting inventory levels, initiating restock orders and even suggesting optimal pricing strategies for hundreds of stores. This can not only reduce overstocking and stock-outs but also improve overall profitability and customer satisfaction.
By focusing on decision intelligence as the end goal, AI orchestration helps bridge the gap between AI capabilities and practical business outcomes.
Benefits of AI orchestration
The key benefit of AI orchestration lies in its ability to enable decision intelligence within an organization. Decision intelligence is the discipline of transforming information into better actions at any scale, effectively automating the full action-to-outcome process.
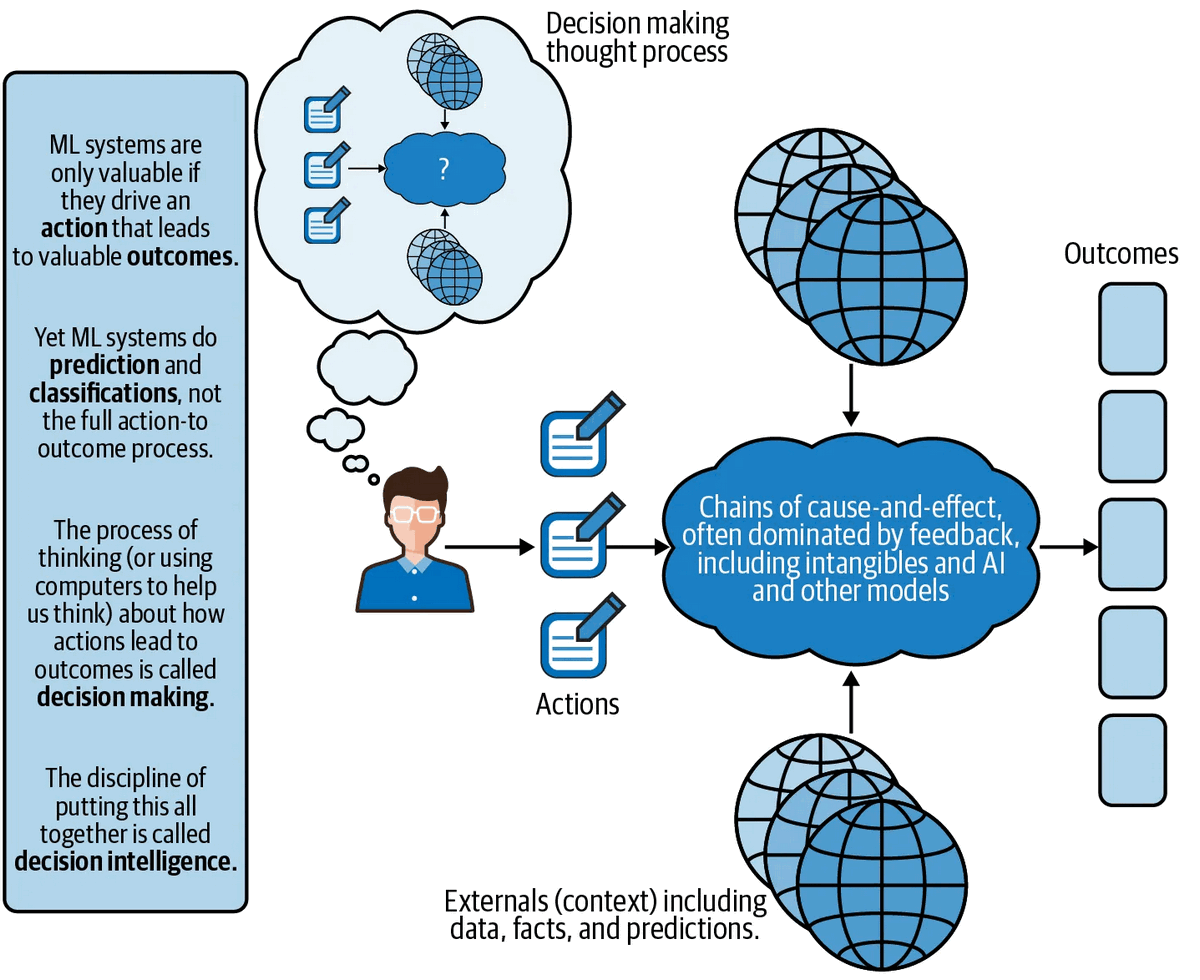
Here's why decision intelligence facilitated by AI orchestration is so important:
- Improved decision-making: By integrating AI into day-to-day operations, companies can make more informed, data-driven decisions at all levels of the business.
- Scalability: Decision intelligence enables better actions not just in isolated instances, but across the entire organization.
- Business alignment: AI orchestration ensures that AI initiatives are directly linked to business outcomes, rather than being isolated technical experiments.
- Operational efficiency: By automating complex decision processes, businesses can work more efficiently and reduce the time and resources spent on routine decisions.
- Adaptability: As AI systems learn and improve over time, the quality of automated decisions can be continuously improved, allowing companies to adapt more quickly to changing conditions.
- Risk mitigation: Proper AI orchestration includes safeguards and oversight, reducing the risks associated with biased or incorrect AI outputs.
- Competitive advantage: Companies that successfully implement decision intelligence through AI orchestration can respond faster and more effectively to market changes and customer needs.
In the following section, we'll explore best practices for implementing AI orchestration to achieve these benefits and enable true decision intelligence in your organization.
Best practices for AI orchestration
Traditional approaches to AI and machine learning projects often started from scratch with raw data. Companies collected huge amounts of information and hoped to gain insights later.
However, this method often did not result in tangible business value.
According to the AI orchestration methodologies report, a more effective approach reverses the process.
Instead of starting with raw data, one should start with clear business goals and work backward. This method, which corresponds to the concept of decision intelligence, focuses on identifying the specific decisions and outcomes you want to improve.
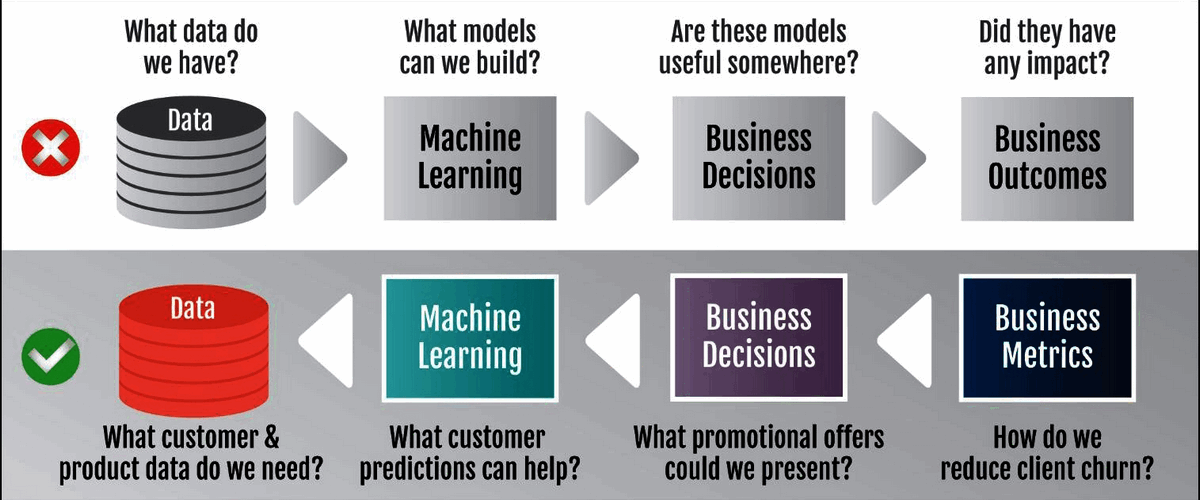
This goal-oriented approach is helpful in several ways:
- It ensures AI projects are aligned with business goals from the start.
- It helps to identify the most relevant data needed for the project and the most suitable ways to use it. AI models may not be even needed in all cases.
- It reduces the risk of bias in AI models by ensuring that the data is representative and contextual.
Other best practices to effectively orchestrate AI projects include:
- Align AI projects with the overall business strategy
- Establish key performance indicators (KPIs) to measure success and manage development.
- Prioritize projects based on their potential impact and feasibility.
2. Choose the right AI orchestration tools
- Assess compatibility with existing infrastructure.
- Evaluate scalability and flexibility to accommodate future growth and changing requirements.
- Ensure support for the required data modalities, such as structured data, images, text and audio, to handle diverse AI applications.
3. Build a robust AI pipeline or solution
- Design a modular and reusable architecture to promote efficiency and consistency across projects.
- Implement rigorous data quality and pre-processing steps to ensure reliable inputs to AI models.
- Establish proper version control and reproducibility not only for the code, but also for the data and models.
4. Monitor and optimize
- Implement real-time monitoring systems to track performance and detect issues promptly.
- Conduct regular audits and reviews to ensure ongoing alignment with business objectives.
- Address model drift and implement retraining strategies to maintain accuracy and relevance over time.
Implementing these best practices requires coordination across the organization. This includes input from front-line employees who understand the current processes, senior managers who set strategic goals, and technical teams who implement the AI solutions.
5 best AI orchestration tools
To implement best practices and effectively orchestrate AI in your organization, you need the right tools.
While the entire process involves much more than just technology, you can significantly optimize your efforts with suitable AI orchestration software. These tools can help manage workflows, integrate AI models into business processes and ensure that your AI initiatives align with your business goals.
Let's take a look at some of the leading AI orchestration tools available today!
n8n
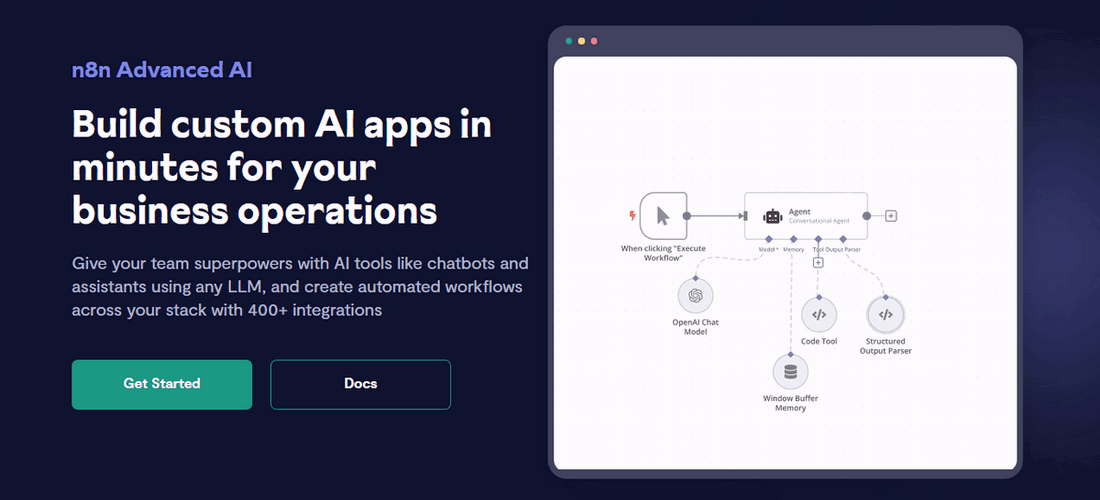
Overview:
n8n is a powerful and flexible workflow automation platform. Unlike specialized ML platforms, n8n can integrate AI directly into business processes.
n8n stands out for its flexible “buy vs. build” approach to AI integration.
It seamlessly connects with ready-made AI tools via API endpoints, enabling rapid prototyping and iteration. As the project grows and the company's AI capabilities mature, n8n makes it easy to switch to in-house AI models, facilitating a smooth transition from third-party services to custom production models.
Key features:
- Intuitive, low-code interface for designing complex AI-powered workflows.
- Pre-built nodes for common AI services, databases and business tools.
- HTTP requests for working with external services, Execute command node for launching internal tools and Code node for writing completely custom code.
- The ability to create completely new nodes for specific AI tasks or proprietary models.
- Powerful tools for pre-processing and manipulating data.
- Designed to efficiently handle complex, multi-step workflows; creation of reusable building blocks for scalability.
- LLM and LangChain support: seamless integration with advanced AI technologies as well as traditional ML models.
- Flexible deployment: options for cloud-based or self-hosted installations that ensure data protection and security.
- Version control and collaboration: features to support team-based development and iteration.
- Error handling and monitoring: maintaining reliable AI-powered processes.
Pricing:
- Free tier for self-hosted community edition with no feature gating
- Cloud plans from $20/month
- Enterprise custom-priced tier for large-scale deployments with advanced support and features
Clear.ml
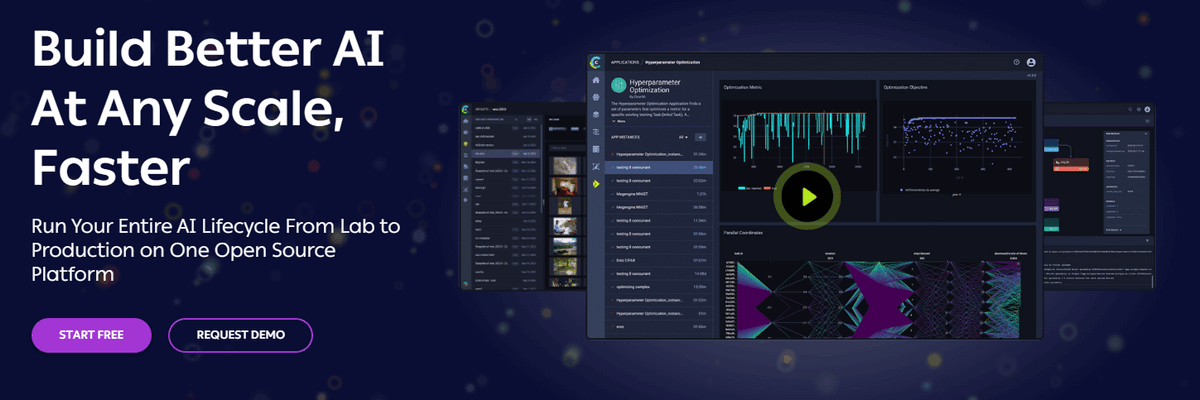
Overview:
Clear.ml is an end-to-end MLOps (DevOps for machine learning) platform for streamlining the entire AI lifecycle. Clear.ml stands out for its "automagical" approach that requires minimal code changes to integrate into existing workflows. The platform is built to support enterprise-grade AI development, offering features like experiment tracking, model management and orchestration.
Clear.ml also offers ClearGPT, a secure enterprise-grade platform for large language models (LLMs) that addresses common challenges like security, performance and data governance. This makes it particularly suitable for organizations that want to leverage generative AI while maintaining control over their data and models.
Key features:
- Experiment manager for automatic tracking of ML experiments.
- MLOps/LLMOps for orchestration and automation of ML/DL/GenAI jobs.
- Data management with version control for datasets.
- Model serving solution for quick deployment of model endpoints.
- Reports module for creating and sharing extensive markdown documents.
- Orchestration dashboard for managing compute clusters.
- Integration with common ML frameworks and development environments.
- ClearGPT for secure, enterprise-grade LLM deployment.
Pricing:
- Free tier for individuals and small teams, includes basic features and 100GB free artifact storage.
- Pro tier: $15 per user/month + usage.
- Even more advanced Scale and Enterprise tiers with custom pricing. Clear.ml also offers a self-hosted open-source core version with a similar to Apache NiFi’s deployment flexibility
Apache NiFi
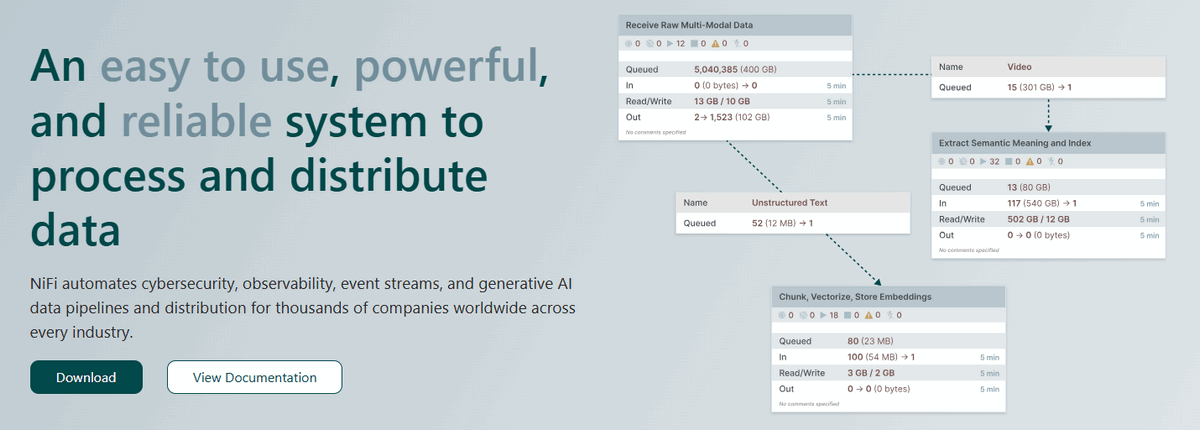
Overview:
Apache NiFi is a powerful and flexible data flow management system. While not as specialized in ML workflows as Clear.ml or Comet.ml, NiFi provides a robust platform for automating the flow of data between systems, making it suitable for managing complex AI pipelines.
NiFi’s visual interface makes it easy to design and modify data flows. This visual approach to creating workflows is similar to Azure Machine Learning’s focus on visual tools. NiFi’s architecture supports high throughput and low latency, making it suitable for processing large amounts of AI data.
Key features:
- Visual creation and management of directed graphs for AI workflows.
- Data provenance tracking for complete information lineage.
- Guaranteed delivery with a persistent write-ahead log.
- Dynamic prioritization of data flows.
- Seamless scaling through clustering.
- Extensive security features, including multi-tenant authorization.
- Flexible extension architecture for custom AI components.
- Site-to-site communication protocol for efficient data transfer between NiFi instances
Pricing:
- Apache NiFi is open-source and free to use. However, organizations may incur costs related to infrastructure, support, and maintenance when implementing NiFi at scale
Comet.ml
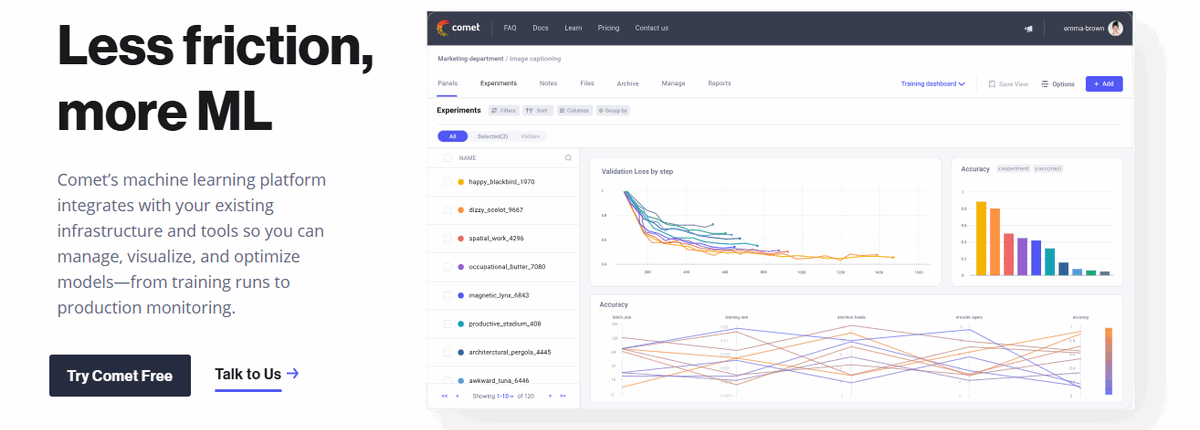
Overview:
Comet.ml is a machine learning platform designed to help data scientists and ML engineers manage, visualize and optimize their models throughout the entire ML lifecycle. Like Clear.ml, it offers robust experiment-tracking capabilities that allow teams to monitor and compare training runs in real time.
Comet.ml’s platform is particularly strong in its support for both traditional machine learning and LLM workflows, similar to Clear.ml and Azure Machine Learning. It provides tools not only for tracking model metrics and hyperparameters, but also for managing and evaluating LLM prompts.
Key features:
- Real-time experiment tracking and visualization.
- Custom dashboard creation for tailored insights.
- Model registry for versioning and deployment management.
- LLM prompt management and evaluation tools.
- Hyperparameter optimization.
- Artifact and dataset versioning.
- Integrates smoothly with popular ML frameworks and can be set up with just a few lines of code.
- Collaboration features for team-based workflows.
Pricing:
- Community: free for personal use, with unlimited projects and 100GB of storage space
- Starter ($50/user/month) for small teams
- Enterprise for larger organizations, custom pricing
Azure Machine Learning
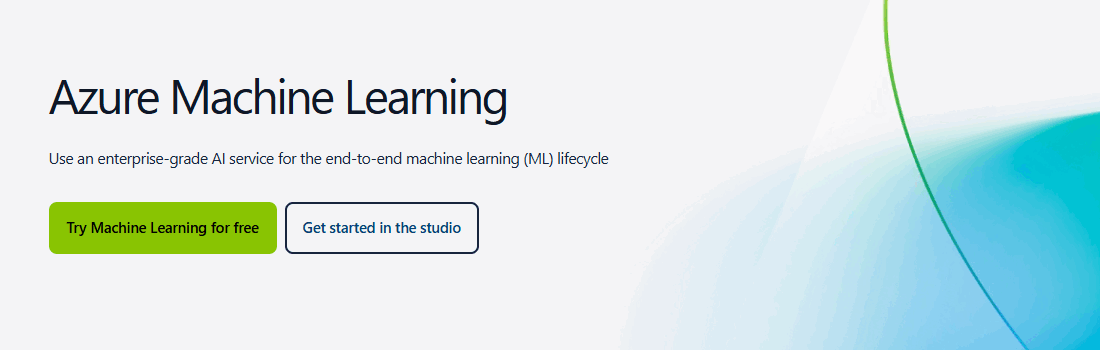
Overview:
Azure Machine Learning is an enterprise-grade service for the end-to-end machine learning lifecycle. Like Clear.ml and Comet.ml, it provides a comprehensive platform for data scientists and developers to build, train and deploy machine learning models. Azure ML supports the full spectrum of machine learning tasks, from data preparation and model development to deployment and management at scale.
The platform integrates well with other Azure services, providing a unique advantage for organizations that have already invested in the Azure cloud ecosystem.
Key features:
- Data preparation with Apache Spark integration.
- Feature store for increased agility in model development.
- Purpose-built AI infrastructure with high-performance GPUs.
- Automated machine learning for rapid model creation.
- Responsible AI tools for interpretability and fairness assessment.
- Model catalog with access to foundation models from various providers.
- Prompt flow for language model workflow design and deployment.
- Managed endpoints for scalable model deployment and monitoring
Pricing:
- Pay-as-you-go: per-second billing without long-term commitments
- Azure savings plan for compute: commit to a fixed hourly rate for 1 or 3 years and get discounted pricing
- Reserved virtual machine instances: commit for 1 or 3 years and get significant discounts for stable workloads
How to use n8n for AI orchestration?
Now that we've explored 5 AI orchestration and automation platforms, let's see one of them in action.
We’ll focus on n8n's AI capabilities as it offers the most flexibility for data handling, custom code integration, and smooth connection of AI services with existing business processes.
Load & transform data
Data preparation is critical to a successful AI implementation. n8n provides powerful tools for extracting, transforming, and loading data from various sources. You can automate simple data loading & conversion processes as well as build complex workflows with dataset comparisons and branching logic.
Run system programs and custom code
The flexibility of n8n enables the smooth integration of custom code and system programs into your AI workflows. With the Execute Command, Code, SSH nodes, you can incorporate proprietary algorithms or specialized tools into AI orchestration processes. n8n serves as a single point for launching all other tools, regardless of whether they are installed on the same system or in the corporate network.
Integrate AI services into business processes
One of n8n's strengths is its ability to connect AI services with existing business tools and workflows.
With the new release of the HTTP Request Tool node, you can connect an LLM to virtually any website or API service with just a single node! Take a look at this in-depth walkthrough with several use cases.
Connect AI insights directly to your operational processes and improve decision-making across the organization. These workflows could be very specific to each company. Therefore, we’ve prepared some simple ideas for your inspiration.
Manage advanced LLM logic on a low-code platform
n8n + LangChain is a powerful combo for the creation of modular AI applications via the intuitive low-code UI. This integration offers a wide range of nodes and tools directly mapped to LangChain concepts:
- Chains and Agents for complex AI logic
- Vector stores for managing embedded data
- Language models from different providers
- Memory nodes for retaining the context of conversations
- Output parsers and text splitters for data processing
- Embedding nodes for text representation
n8n empowers both technical and non-technical users to create sophisticated AI workflows.
Orchestrate hybrid AI environments
n8n excels at managing workflows that combine cloud-based AI services with on-premises custom models. This hybrid approach enables companies to balance the benefits of existing AI platforms with the need for data privacy and custom-built AI solutions.
These use cases show how n8n can adapt to the different stages of an organization's AI journey, from initial experimentation with ready-made services to deploying custom, in-house models.
Wrap Up
In this article, we've looked at AI orchestration in modern business operations and the strategies to improve the success rate of AI deployments.
We’ve covered various specialized AI orchestration tools as well as n8n and highlighted its features in integrating AI into business processes.
Finally, we’ve also demonstrated practical applications of n8n for AI orchestration, from data preparation to managing hybrid AI environments.
What’s next?
Here are some next steps to start your AI orchestration with n8n:
- Expand your AI toolkit by checking out our article on the best AI coding assistants.
- Check out our guide to building intelligent ERP solutions with n8n.
- If you're a developer, don't miss our handy guide on AI agents.
Choose from our cloud plans and create a trial n8n account today. Remember, whether you're just beginning or advancing your AI journey, n8n provides the flexibility and power to meet your needs.